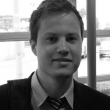
CFD Consultant, EnginSoft Nordic
M.Sc. Ragnar Skoglund (Master in Space Engineering, Aerospace at Lulea University of Technology) is working as a CFD consultant at EnginSoft Nordic. He has a great passion for the aerospace industry and has been involved in several student projects in this field. He has been studying space related courses above the polar circle in Kiruna, Sweden, and holds a student license in paragliding. Ragnars expertise field within CFD is: External and internal flows, turbomachinery, airfoils and design optimizating in modeFRONTIER. Past experience in CFD main includes external flow on a small UAV aircraft and aerodynamic performance uncertainty of a space turbine (Master Thesis).
Speeches
Material design involves many uncertainty sources. At Volvo Car Corporation engineers recognized the importance of assessing the variation in material quality and storing all the collected data in a way that enables easy access and reuse.
This presentation tray to address two main issues: how should material variations be controlled in simulations, optimizations and robustness analyzes? How should test data and statistics be stored in order to sample suitable curves for simulations? The proposed approach aims at capturing and characterizing the set of stress-strain curves relevant for the material of interest and made them available for efficient reuse in CAE models. In order to assure easy knowledge extraction, such variation representation should be very accurate, compact, and flexible.
modeFRONTIER has been successfully used to calibrate the material model against a set of test data curves to capture the variation in material properties. The test data consist of 25 tensile strength tests obtained from SSAB, done for 25 species from 25 different rolls. A subproject including the Curvefitting Node is used to calibrate the model parameters by minimizing the mean square error between the test data curve and the material model.
modeFRONTIER main project collects then all calibrated model parameters for each curve.
Thanks to the correlation analysis between model parameters linear dependencies and monotonic behavior are discolsed. When the calibration is finished it is of interest to sample a dataset of curves from the material database which have the same stochastic properties as the SSAB test dataset. This can be achieved by performing a Monte Carlo or Latin Hyper cube sampling in the domain of variation. To obtain a statistical confidence a stepwise increased data set can be used to disclose when the standard deviation of material properties converges with the data set size.